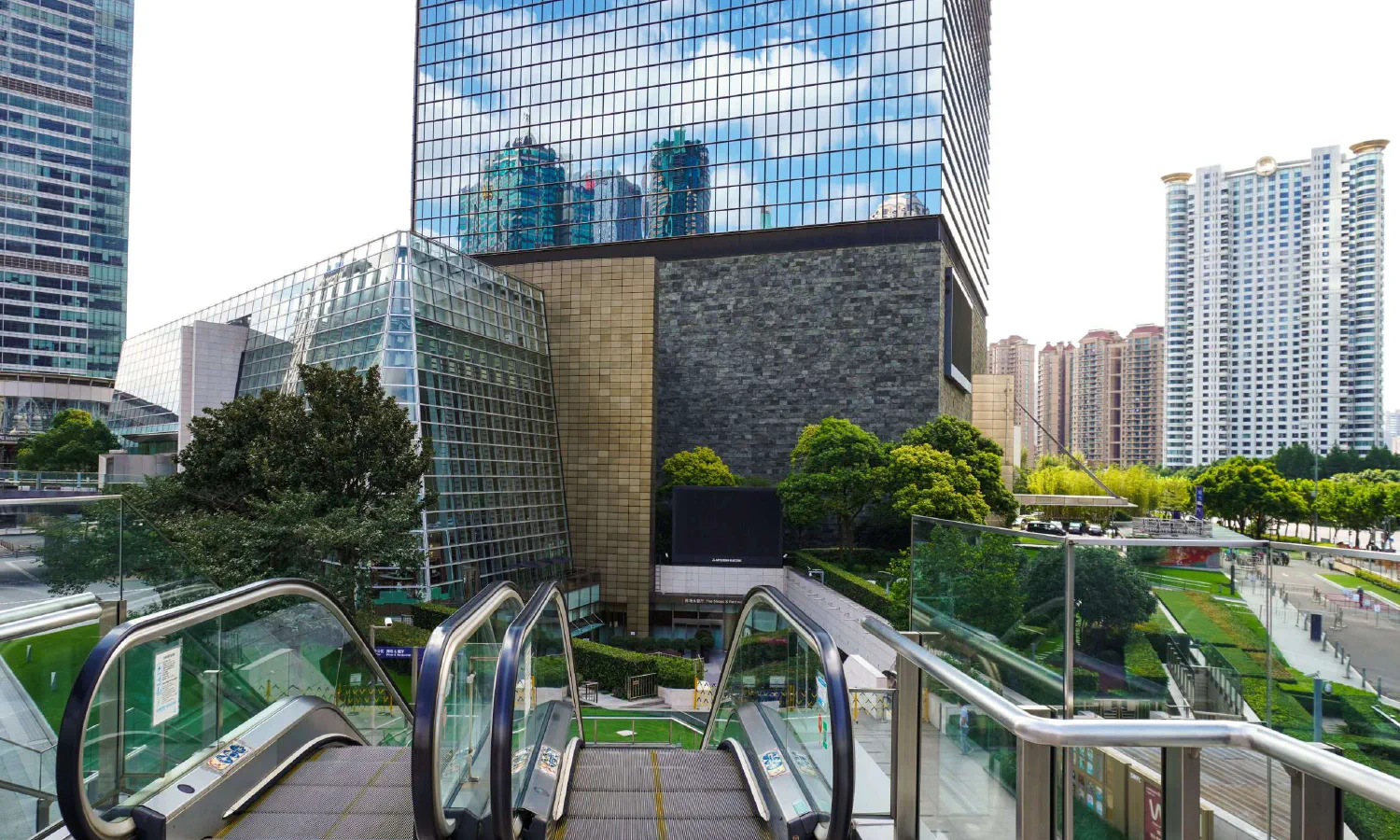
Revolutionizing Investing with AI: Towards Smarter Stock Market Choices
In recent years, Artificial Intelligence (AI) has rapidly changed various industries, and finance is no exception. AI-powered investment strategies and platforms are evolving everyday, providing institutions and private investors more effective ways to grow their wealth. In this article, we will take a deep dive into the how and why is this possible.
Understanding Artificial Intelligence (AI) and Machine Learning (ML)
Artificial Intelligence encompasses a broad range of technologies that enable machines to perform tasks typically requiring human intelligence, such as visual recognition, natural language understanding, decision making, problem-solving or learning from experience. Machine learning is a subset of technologies within the Artificial Intelligence (AI) domain. ML is that allows software applications to become more accurate in predicting outcomes without being explicitly programmed to do so. To achieve that, Machine Learning algorithms use past and/or present data to construct models that capture the relationships between inputs and outputs, enabling accurate predictions for new (or unseen) inputs.
ML empowers investment firms to refine their decision-making processes and enhance performance significantly. According to this report from J.P. Morgan Asset Management, ML-based investment strategies aim to consistently deliver uncorrelated alpha streams while adapting to shifting market conditions.
Some of the most successful firms such as Renaissance Technologies, Two Sigma Investments or Bridgewater Associates have successfully integrated ML across various aspects of investing, including data analysis, portfolio optimization, risk management, and trading execution (source: Investing News).
A report by Cerulli shows that AI-led hedge funds produced cumulative returns of 34 percent in the three years through May 2020, compared with a 12 percent gain for the overall hedge fund universe (source: Institutional Investor).
So… How does ML works under the hood?
Machine learning models are essentially statistical mathematical models that identify patterns within data. In simpler terms, imagine a linear regression equation: y = Ax + B. This equation establishes a relationship between an input variable (x) and an output variable (y) through coefficients A and B. In the context of financial markets, x could represent historical price data and technical indicators, while y could represent future price movements.
However, the true relationship between past and future prices is often complex and not easily captured by a single equation. This is where ML comes in. By analyzing vast amounts of historical market data (x-y pairs), ML algorithms can learn the underlying, non-linear relationships between various factors and stock prices. The training process involves optimizing the model’s internal parameters to minimize the prediction error for the data it’s trained on. These parameters can number in the hundreds of thousands, or even millions, for complex models.
The ultimate objective of any ML model is to achieve strong generalization power. This translates to the ability to accurately predict future outcomes (y) for unseen data points (i.e., new price movements) with minimal error. In the context of stock markets, this translates to making predictions for future price movements based on historical data and current market conditions, even for situations the model hasn’t encountered before.
And… why can this be useful to investors?
Before placing their money, many investors research companies’ financial data and news, compare them to industry peers, analyze technical indicators, and consider the macroeconomic context. Some seek advice from analysts or financial experts to inform their decisions. However, this process is complex and time-consuming, limiting the number of companies that can be thoroughly evaluated. This complexity often results in a focus on only a few key factors. Moreover, the opinions of expert financial analysts can be opaque, raising questions about fairness and potential biases or interests influencing the analysis. Investors may wonder if they themselves are unconsciously affected by instincts or emotions negatively impacting their investment choices.
This is where Machine Learning offers advantages:
- Research at Scale: The models can handle vast amounts of data from thousands of companies over several years. Analyzing this much data would be highly difficult, if not impossible, for an average investor/analyst, even with strong financial and mathematical skills.
- Complexity: The models can work with a large number of variables (combining fundamental, technical, and contextual/macroeconomic data) and leverage complex relationships to improve predictions. This can reveal insights and patterns that would be incredibly difficult, even for financial experts, to identify using a purely theoretical approach.
- Knowledge Discovery: While incorporating expert knowledge is possible, these models can also perform well without a priori knowledge or rules. They extract relationships and knowledge from the training data, “learning” to focus on the most relevant variables and ignore the rest.
- Objectivity: These algorithms do not factor in biases, interests, opinions, or emotions. Their results are strictly the outcome of the chosen algorithm, parameters, data, and training method. At ML Alpha, every single score, rank, or rating is purely data-driven (or data-based). This means they are the output of checks based on data, rules, or machine learning models, free from opinions, emotions, or vested interests.
But…. What are the limitations?
AI can leverage advanced algorithms to analyse thousands of financial assets and identify the ones that fit best with the investor’s goals and risk tolerance. It results in an intelligent mix that significantly boosts your short/long-term performance. Create a Portfolio from scratch or optimise an existing one using our AI Portfolio Management tool.
If you wish to know more about how our AI Algorithms are working you can deep dive in our AI Investing article.
So, in conclusion…
The adoption of AI and Machine Learning technology has revolutionized the landscape of stock market trading and investing. As showcased through the success stories of hedge funds and investment firms, these advanced algorithms have proven their ability to analyze vast amounts of data, uncover complex patterns, and make informed predictions, ultimately leading to significant returns on investment. Looking ahead, the future of investing holds even more promise as AI and Machine Learning continue to evolve, offering innovative solutions and strategies to navigate the ever-changing market dynamics.
Furthermore, while currently predominantly utilized by large financial institutions and hedge funds, there is a growing effort, exemplified by initiatives like ML Alpha, to democratize access to Machine Learning-driven investment strategies. By making these technologies more accessible to a broader range of investors, we aim to empower individuals and smaller firms with the tools necessary to compete and thrive in the dynamic world of finance.
Thanks a lot for reading this article, as always we really value our customers/readers feedback. You can request features of anything on our Feedback Page. You can get started with ML Alpha for free here.